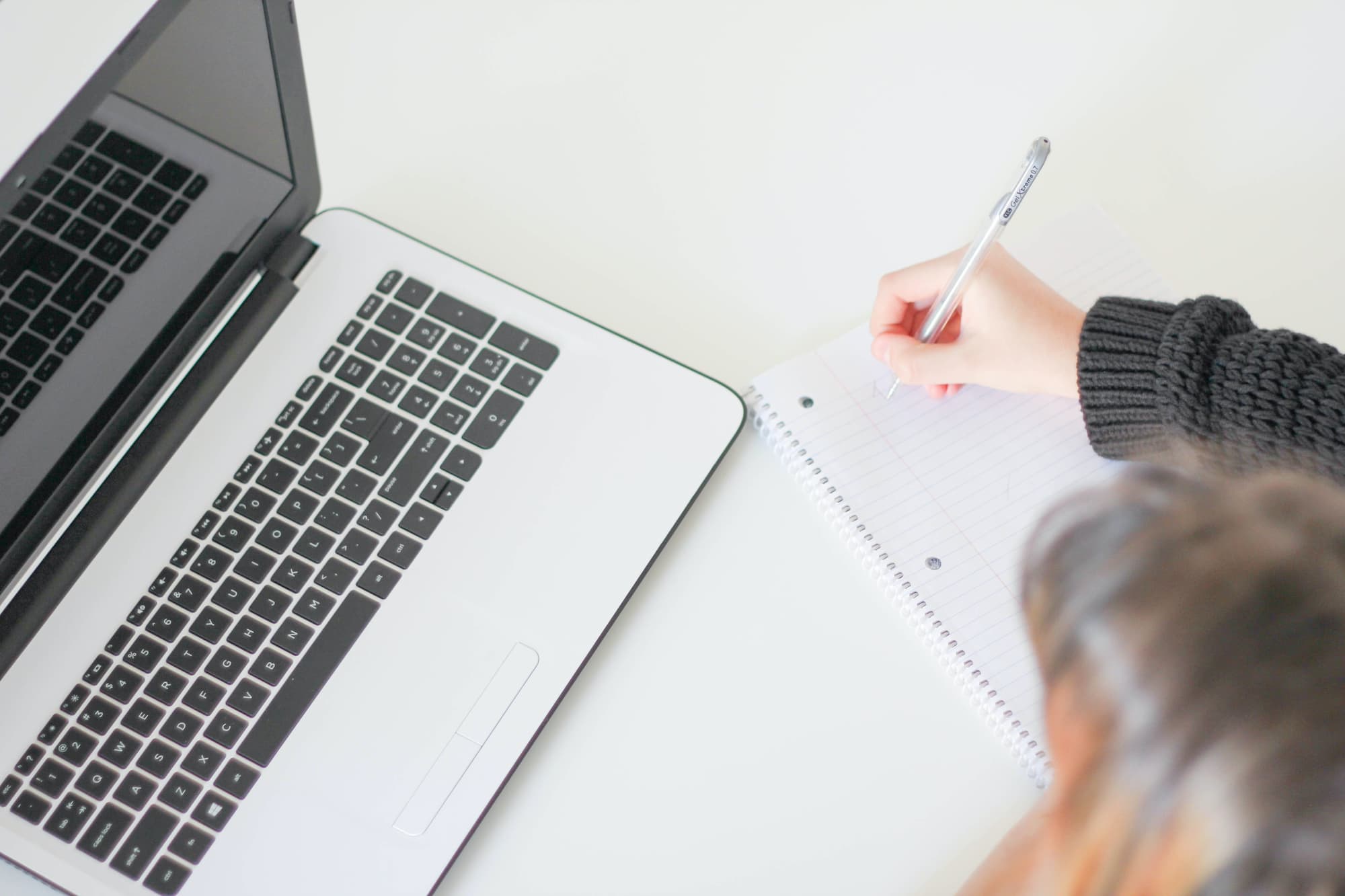
Performance evaluation plays a pivotal role in assessing the effectiveness of investment programs. It is bifurcated into two primary components: performance measurement and performance attribution. Performance measurement quantifies the overall results of an investment program, both in absolute terms and relative to various benchmarks, with or without suitable adjustments for risk. Conversely, performance attribution involves a more detailed analysis, breaking down the portfolio into sub-portfolios or economic exposures, and re-aggregating these individual analyses to identify the sources of return and the strengths and weaknesses of the investment process.
Conducting these analyses is not straightforward due to the high level of noise in financial markets. For instance, the standard deviation of most financial market returns is higher than their mean return, a level of noise surpassing that associated with deep-space communication. Consequently, performance evaluation has developed numerous tools and techniques, often specialized and attuned to the needs of specific types of investments.
Performance analyses and attributions should be reported to clients regularly, often quarterly, but at least once a year. These reports should be in a clear, simple format that encourages conversation, discussion, and debate, often with the manager in attendance.
Performance measures based on return are essential tools in the world of finance, used to evaluate the performance of a portfolio against a benchmark. These measures are calculated in accordance with the Global Investment Performance Standards (2020). For instance, if we consider the S&P 500 as a benchmark, we can evaluate the performance of a mutual fund portfolio against it.
The total return of a portfolio in a given period, denoted by \(r_{P, t}\), includes the impact of price changes and cash flows such as coupon and dividend payments. For example, if a portfolio consists of stocks that have appreciated in value and paid dividends, both these factors would contribute to the total return.
The arithmetic active return, denoted by \(R_{A, t} = R_{P, t} – R_{B, t}\), shows the difference between the portfolio’s return and the benchmark’s return. This measure helps to understand how much the portfolio has outperformed or underperformed the benchmark.
The excess return, denoted by \(R = R_{P, t} – R_{f, t}\), shows the difference between the portfolio’s return and the risk-free return. This measure helps to understand the additional return earned by taking on risk as compared to a risk-free investment like government bonds.
The logarithmic active return and logarithmic excess return, denoted by $$R˜_{A, t} = ln (1 + R_{P, t}) – ln (1 + R_{B, t})$$ and $$R˜_{E, t} = ln (1 + R_{P, t}) – ln (1 + R_{f, t})$$ respectively, take into account the impact of compounding. These measures provide a more accurate picture of returns over multiple periods.
Risk-adjusted measures measures amalgamate a return measure and a risk measure into a single performance indicator. The most commonly used risk-adjusted performance measures are the Sharpe Ratio and the Information Ratio. The Sharpe Ratio is the ratio of the average excess return to the standard deviation of the excess return, while the Information Ratio is the ratio of the average active return to the standard deviation of the active return. These ratios are beneficial for ranking portfolios, with investors generally favoring portfolios with high Sharpe and Information Ratios.
The Sharpe Ratio can be interpreted as a measure of the likelihood that the portfolio’s return surpasses the risk-free rate or the return of its benchmark. Logarithmic returns offer advantages when measuring performance and when computing risk-adjusted measures of performance such as the Sharpe Ratio and the Information Ratio.
The Logarithmic Sharpe Ratio is calculated as:
$$ \text{Logarithmic Sharpe Ratio} = \frac{\text{Mean (Excess logarithmic return)}}{\text{Standard deviation (Excess logarithmic return)}}$$
The Logarithmic Information Ratio is calculated as:
$$ {\text{Logarithmic Information Ratio}} = \frac{ {\text{Mean} \\ \text{(Active logarithmic return)}}}{{\text{Standard deviation} \\ \text{(Active logarithmic return)}}}$$
Risk can be encapsulated in ways that reflect the asymmetry of returns. Popular downside risk measures include VaR, ES (also known as cVaR or conditional VaR), and Semi-standard Deviation. VaR is a percentile of the loss distribution of an asset or a portfolio. ES is the expected loss in only those periods when VaR is exceeded. Semi-standard Deviation is the downside analogue of standard deviation.
The Sortino Ratio is calculated as:
$$ \text{Sortino Ratio} = \frac{\text{Mean (Return) – MAR}}{\text{Semi-Deviation (Return – MAR)}}$$
The Logarithmic Sortino Ratio is calculated as:
$$ {\begin{align*} & \text{Logarithmic} \\ & \text{Sortino Ratio} \end{align*}}= \frac{ { \text{Mean (Logarithmic return)} – \text{Logarithmic MAR}}}{\text{Semi}-\text{Deviation (Logarithmic return – Logarithmic MAR)}}$$
The Logarithmic Sharpe and Information Ratios are useful baselines to start an analysis of a portfolio, and their downside risk-based analogues such as the Sortino Ratio and the Logarithmic Sortino Ratio are useful supplements. These performance measures are best applied to liquid investments that are priced continuously in well-functioning capital markets. If applied to illiquid priced portfolios, their values will likely be overstated, as the measure of risk in their denominators will be underestimated. These measures are usually computed using monthly returns and are approximately annualized by multiplying them by \(\sqrt{12}\).
Performance attribution is a critical process in portfolio management, which helps in understanding the factors contributing to the performance of a portfolio. It involves breaking down and projecting a portfolio’s return along relevant axes, such as sectors or individual securities. The choice of these axes is as crucial as the choice of benchmark for performance measurement. The most commonly used attribution model is the Brinson–Hood–Beebower (1986) additive or accounting-based decomposition.
Under the Brinson, Hood, and Beebower model, two auxiliary portfolios are created – an allocation effect portfolio \((\textit A)\) and a selection effect portfolio \((\textit S)\). The allocation effect portfolio \((\textit A)\) captures the impact of overweighting sectors that perform well and underweighting sectors that perform poorly. For instance, if a portfolio manager decides to allocate more funds to the technology sector anticipating a boom, this decision’s effect is captured by the allocation effect portfolio. On the other hand, the selection effect portfolio \((\textit S)\) captures the impact of overweighting individual securities that perform well and underweighting individual securities that perform poorly. For example, if a portfolio manager decides to invest more in Apple Inc. shares expecting a rise in its stock price, this decision’s effect is captured by the selection effect portfolio.
The returns of portfolios P, B, \(\textit A\), and \(\textit S\) in a given period are calculated as follows:
$$r_t^P = \sum_{i} w_{i, t-1}^P \times r_{i, t}^P$$
$$r_t^B = \sum_{i} w_{i, t-1}^B \times r_{i, t}^B$$
$$r_t^{\textit A} = \sum_{i} w_{i, t-1}^P \times r_{i, t}^B$$
$$r_t^{\textit S} = \sum_{i} w_{i, t-1}^B \times r_{i, t}^P$$
The allocation effect (A) and the selection effect (S) are defined as follows:
$$A = r_{\textit A} – r_B$$
$$S = r_{\textit S} – r_B$$
However, the allocation and selection effects do not add up to the active return of the portfolio. There is a third term, the interaction effect (I), which bridges this gap. The interaction effect is the extra return earned by overweighting sectors in which the selected assets outperform their sector and underweighting sectors in which the selected assets underperform their sector. It is calculated as follows:
$$I = r_P – r_{\textit A} – r_{\textit S} + r_B$$
The Brinson–Fachler (1985) attribution simplifies the Brinson–Hood–Beebower scheme by folding the interaction term into the selection term. Each term in an attribution is referred to as the contribution from a specified source. For example, the allocation effect is referred to as the contribution from allocation.
Factor-Based Attribution is a technique employed by financial analysts to calculate attributions when comprehensive breakdowns of portfolio holdings and returns are not readily available. This is accomplished by correlating the portfolio’s returns with the returns of a relevant set of factors. The attributions vary depending on the factor model used.
Fixed income factors and equity factors typically differ, with one exception. For example, if the Aa spread factor (the difference in return between Aa-rated bonds and Treasury bonds, such as those issued by the US government) increases by 1 standard deviation, the active return of the fixed income portfolio is expected to increase by 0.35 standard deviations. Conversely, if the difference in return between value stocks (like Berkshire Hathaway) and growth stocks (like Amazon) increases by 1 standard deviation, the active return of the equity portfolio is expected to decline by 0.56 standard deviations.
The fixed income portfolio has a higher exposure to high-quality bond returns than its benchmark during the analysis period, while the equity portfolio has a higher exposure to growth stock returns than its benchmark during the same period.
Both accounting and factor-based attributions provide wealth managers with valuable insights into the sources of a portfolio’s returns. This enables them to evaluate whether a manager’s actual investment process aligns with their stated investment philosophy. Attributions also allow portfolio managers to contemplate future factor behavior and how they might want to position their portfolios to capitalize on their predictions.
The statistical significance is vital in financial markets, especially in portfolio management. It aids in determining the true mean of a random variable and in identifying whether a portfolio manager is underperforming or not.
The t-statistic is a statistical measure used to ascertain if the true mean of a random variable is greater than 0. It is calculated using the formula:
$$\text{t-statistic} = \frac{\text{Sample mean}}{\text{Sample standard deviation}} \times \sqrt{N}$$
Where:
A t-statistic of 2 roughly translates to a 95% probability that the true mean of the return distribution is positive. For example, if the return of a portfolio managed by Berkshire Hathaway is 10% per annum, and its standard deviation is 20% per annum, we need more than 16 years for the t-statistic to exceed 2. This exceeds the length of most careers at a single firm, emphasizing the need for comprehensive performance and attribution analyses.
Performance and attribution analyses must be continually updated and monitored to ensure rapid detection of problems. Clients must maintain an active and ongoing dialogue with their portfolio managers to understand the link between various analyses and the state of the investment processes.
The CUSUM (CUmulative SUM) is a Statistical Process Control scheme used to monitor a portfolio’s performance and attribution components. It raises an alarm when sufficient statistical evidence of underperformance accumulates. The CUSUM detects underperformance faster than any other algorithm for any given level of false alarms. It takes about 3.5 years to confidently identify an underperforming manager, not the 16 years as suggested by the t-statistic calculation.
Once underperformance has been identified, a thorough and impartial investigation into the reasons for it must be launched. If a causal explanation for the underperformance is found, it should be correlated with the manager’s stated investment philosophy and process to determine if it was caused by a flaw in the investment process or a deviation from it. A course of action should be decided upon, ranging from no action at all to termination. The results of the study should be shared with the manager so that their investment process can improve.
The process of manager selection involves a series of steps to ensure the chosen manager can effectively meet the investment goals and risk tolerance of the client.
Practice Questions
Question 1: Which of the following statements is true regarding the challenges in performance evaluation?
- Performance evaluation is straightforward due to the low level of noise in financial markets.
- The process of performance evaluation is made easier due to the high level of noise in financial markets.
- The high level of noise in financial markets makes the process of performance evaluation more difficult, necessitating the development of specialized tools and techniques.
Answer: Choice C is correct.
The high level of noise in financial markets makes the process of performance evaluation more difficult, necessitating the development of specialized tools and techniques. The term “noise” in financial markets refers to the high level of volatility and unpredictability, which makes it difficult to accurately assess the performance of an investment. This is because the standard deviation of most financial market returns is higher than their mean return, which means that the returns are highly dispersed and can vary significantly from the average return. This high level of noise makes it difficult to distinguish between random fluctuations and genuine trends, making the process of performance evaluation more complex and challenging. As a result, many specialized tools and techniques have been developed to help in the performance evaluation process. These tools and techniques are designed to filter out the noise and identify the underlying trends, thereby providing a more accurate assessment of the performance of an investment.
Choice A is incorrect. Performance evaluation is not straightforward due to the high level of noise in financial markets. The high level of volatility and unpredictability in financial markets makes it difficult to accurately assess the performance of an investment, necessitating the use of specialized tools and techniques.
Choice B is incorrect. The high level of noise in financial markets does not make the process of performance evaluation easier. On the contrary, it makes it more difficult by increasing the level of uncertainty and unpredictability, making it harder to distinguish between random fluctuations and genuine trends.
Question 2: Performance evaluation is a complex subject and cannot be fully covered within one portion of a single reading. It is crucial that performance analyses and attributions be reported to clients regularly, often quarterly, but at least once a year. These reports should be in a clear, simple format that encourages conversation, discussion, and debate, often with the manager in attendance. How often should performance analyses and attributions be reported to clients?
- Performance analyses and attributions should be reported to clients annually.
- Performance analyses and attributions should be reported to clients monthly.
- Performance analyses and attributions should be reported to clients regularly, often quarterly, but at least once a year.
Answer: Choice C is correct.
Performance analyses and attributions should be reported to clients regularly, often quarterly, but at least once a year. This is because performance evaluation is a complex subject and requires regular updates to ensure that clients are kept informed about the performance of their investments. Regular reporting, often on a quarterly basis, allows for timely feedback and adjustments to be made if necessary. It also provides an opportunity for clients to ask questions and engage in discussions with the manager, thereby promoting transparency and trust. Furthermore, regular reporting helps to ensure that clients are not surprised by any sudden changes in the performance of their investments. Therefore, while annual reporting is the minimum requirement, more frequent reporting is often beneficial and is considered best practice in the investment management industry.
Choice A is incorrect. While performance analyses and attributions should be reported to clients at least once a year, this is the minimum requirement and not the ideal frequency. More frequent reporting, often on a quarterly basis, is considered best practice in the investment management industry.
Choice B is incorrect. Monthly reporting of performance analyses and attributions is not mentioned in the question. While more frequent reporting is generally beneficial, monthly reporting may not be practical or necessary in all cases. The ideal frequency of reporting depends on various factors, including the nature of the investments, the needs and preferences of the clients, and the resources available to the manager.
Private Wealth Pathway Volume 1: Learning Module 4: Investment Planning; LOS 4(d): Evaluate the success of an investment program for a private client based on portfolio reporting and review