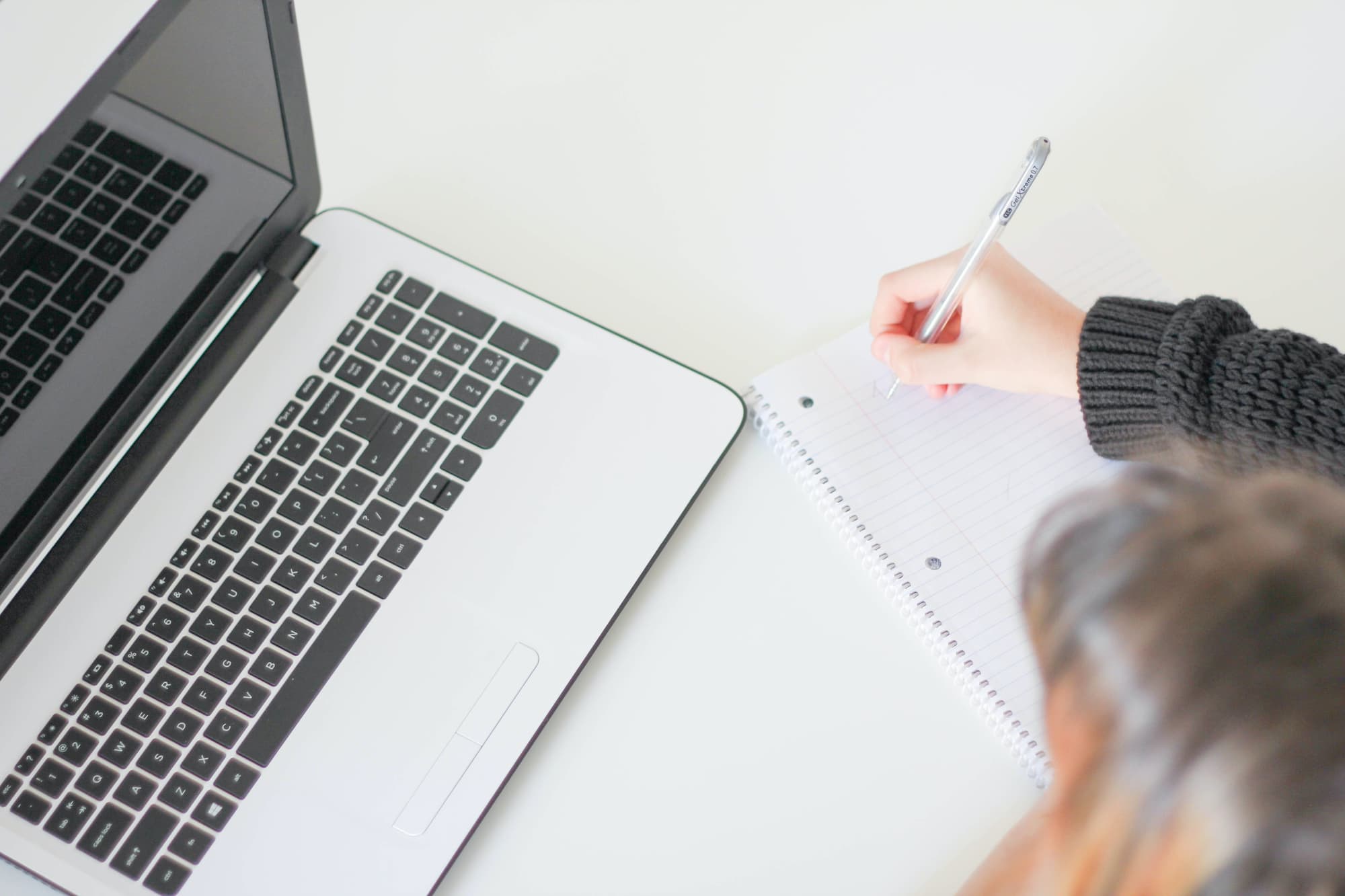
Private clients can invest in both public and private markets. However, this discussion primarily focuses on public markets due to the complexity of modeling returns for private investments, which often have intricate fee structures including base fees, performance fees, and high-water marks.
Despite the complexity of modeling returns for private investments, estimating their risk is relatively straightforward. For instance, the risk of a private equity fund like Blackstone Group can be estimated using various financial models.
The impact of taxes on returns is a crucial factor when forming capital market expectations, which are used as inputs in the construction of optimal portfolios.
Understanding a client’s risk tolerance is a crucial aspect of wealth management. This involves comprehensive discussions to understand the client’s goals, constraints, fears, and desires. However, the effectiveness of risk tolerance questionnaires in accurately characterizing investor behavior, especially during stressful times, is questionable. This necessitates significant judgment from a wealth manager.
Academic studies, such as the one conducted by Sivarajan in 2018, have pointed out a high degree of subjectivity in the client questionnaire approach. This subjectivity can potentially increase the influence of the wealth manager’s own views on risk in making investment decisions for a client. For example, a wealth manager might be more conservative or aggressive in their investment strategy based on their personal risk tolerance, which could influence their recommendations to clients.
Furthermore, research by behavioral economists, like Thaler in 2015, has shown that investors’ responses can be influenced by the framing of a question. For instance, a question about a small dollar loss on a small portfolio may elicit a different response than a question about a significant dollar loss on a large portfolio, even if the percentage losses are identical. This highlights the importance of carefully crafting questions to accurately gauge a client’s risk tolerance.
As part of the wealth management process, understanding a client’s risk tolerance is crucial. This can be achieved through various methods, including direct conversations with the client, which can provide valuable insights that may not be evident from standard risk tolerance questionnaires or personality assessments.
The wealth manager plays a crucial role in understanding and educating the client about investment risk. This involves creating an investment profile based on the client’s risk tolerance, which guides the choice of suitable investments.
Risk Tolerance with Multiple Goals revolves around the understanding that a client’s risk tolerance varies across their different financial objectives. For example, a client might be more risk-averse when it comes to short-term goals like funding their child’s education, but more risk-tolerant when it comes to long-term goals like retirement planning.
This variation in risk tolerance poses a unique challenge for wealth managers. To address this, they can employ a strategy of dividing the client’s portfolio into several ‘Mental Accounts’. Each account represents a specific goal – for instance, one for education, another for housing, and another for retirement.
Each mental account is then allocated a sub-portfolio that reflects the client’s risk tolerance for that specific goal. When these sub-portfolios are combined, they form the client’s total portfolio. This strategy allows for a more personalized management of the client’s portfolio, considering the varying risk tolerances for different goals.
The journey of portfolio construction commences with the crucial step of formulating capital market assumptions. This step involves estimating the expected return and risk profile of various capital markets where the investor is likely to participate. This process is undertaken post the identification of the client’s risk profile and prior to the construction of a portfolio that mirrors their needs.
However, the formulation of capital market assumptions is often executed haphazardly. It’s not uncommon for assumptions about prospective expected returns to be formulated by extrapolating historical realized returns. This approach can be perilous during periods of extreme exuberance or distress, such as just before the technology bubble burst in March 2000 or during the depths of the Great Financial Crisis in March 2009.
Estimating the expected return of high-grade fixed-income indices with minimal default risk is a relatively straightforward task. This is due to a theorem proved in Langetieg, Leibowitz, and Kogelman (1990) which states that over a period equal to twice its modified duration, the total return of a default-free constant-duration bond index is well approximated by its initial yield. This theorem is illustrated by the range of returns for one- and five-year constant-duration bond indices over various holding periods when interest rates follow a random walk with a volatility of 150 bp/annum.
For short-duration indices and cash, the path of future interest rates is hard to predict, and it is common to use the current yield to maturity (YTM) to estimate expected returns. The central insight here is that the returns to cash should roughly mirror inflation over the long term.
When dealing with high-yield indices, both the probability of default and loss given default must be considered.
The expected return of an index is given by:
$$ER_{\text{Index}} = YTM_{\text{Index}} – (DR_{\text{Index}} \times LGD_{\text{Index}})$$
Where:
However, these expressions are approximate because they do not consider index construction rules that govern when bonds enter and leave indices, changes in interest rates, and the shape of the yield curve. Such details are beyond the scope of this reading.
Sometimes, a new fixed-income market segment arises that necessitates rethinking expected returns from the ground up. This was once true of mortgages and structured securities and is now true of private credit. Investors will need to review deals, prospectuses, covenants (which have changed dramatically over the past three decades), and issuance patterns to determine how these securities might perform in good times and bad.
Estimating the expected return of equity indices is a challenging task, more so than estimating the expected return of fixed-income indices. This complexity arises because equity investors capture a portion of the growth in corporate earnings, a factor that must be considered when estimating the expected returns of equities.
For instance, according to Bernstein’s and Arnott’s 2003 paper titled “Earnings Growth: The 2% Dilution,” per-share corporate earnings and dividends tend to grow at a slower pace than the economy. This is due to the dilutive effect observed in every country other than Sweden from 1900 to 2000, even in countries that were not affected by war.
Further research by L’Her, Masmoudi, and Krishnamoorthy in 2018, titled “Net Buybacks and the Seven Dwarfs,” builds on Bernstein and Arnott’s (2003) research. Both articles focus on dividends instead of earnings, as there is more data available on dividends worldwide than on earnings.
Understanding the growth of corporate earnings and dividends per share is crucial for investors. However, various dilutive mechanisms can slow this growth. The primary mechanism is the division of economic growth between the existing publicly traded economy and the new economy. This dilution impacts expected returns by reducing the growth rate of dividends, a key component in the calculation of expected returns.
In 2023, the CFA Institute’s Research Foundation published the monograph Revisiting the Equity Risk Premium, which can be a valuable resource for understanding and estimating expected returns.
$$\text{Expected Return} = \text{Forward Dividend Yield} + \text{Growth Rate of Dividends}$$
‘Expected Return’ is the return that an investor anticipates on an investment. ‘Forward Dividend Yield’ is a prediction of a company’s dividend yield over the next 12 months. ‘Growth Rate of Dividends’ is the annualized percentage rate of growth that a particular stock’s dividend undergoes over a period of time.
When it comes to evaluating the potential return on investment, two approaches stand out: the Cyclically Adjusted Price Earnings Ratio (CAPE) and Reverse Engineering the Dividend Discount Model. Both methods provide valuable insights into expected returns and can be used to make informed investment decisions.
Conceived by Professor Robert Shiller, CAPE is a measure of the current price of an equity index relative to the 10-year average of its real (CPI-adjusted) earnings. For instance, if we consider the S&P 500 index, a high CAPE value would suggest lower expected returns and vice versa. This data can be accessed on Professor Shiller’s website at Yale University.
This method is used to deduce the expected return. It employs a variant of the Gordon Growth Model, using estimates of dividend growth derived from estimates of earnings growth. A useful resource for this approach is the website http:// www . market -risk -premia . com, which publishes long-term estimates of expected return for several countries.
Estimating the expected return of asset classes such as equities and fixed income can be complex due to the unavailability of data. Two primary methods are generally employed. The first method uses first principles. For example, the expected return for timber can be estimated using biological growth, while the relationship between spot and futures prices can be used for commodities.
The second method involves adjusting risk premia to baseline estimates of expected returns for asset classes that can be reasonably mapped to other asset classes. For instance, the expected returns for private equity and private credit can be estimated using the expected returns for stocks and bonds.
In cases where no information is available, such as for collectibles and art, analysts may resort to using historical estimates. However, these estimates may be biased and may not accurately represent the expected returns.
Asset class risk is typically estimated by its variance and covariance with other asset classes. For instance, the risk of investing in the technology sector can be estimated by its variance and its covariance with other sectors like healthcare or finance. Analysts often use about five years of monthly returns to estimate a covariance matrix, which is then adjusted using a statistical technique known as shrinkage to reflect future predictions.
Shrinkage involves computing a sample covariance matrix using all available data, extracting a set of estimates of security standard deviations and a correlation matrix, and then calculating the weighted average of this sample correlation matrix and the identity matrix. This process is akin to adjusting the historical correlation data with a neutral baseline (the identity matrix) to make future predictions. The shrunk correlation matrix is then multiplied appropriately by the estimates of security standard deviations to compute a shrunk covariance matrix.
A five-year lookback is often used as it strikes a balance between focusing on the immediate past, which is likely to better reflect risk in the near term, and all available history, which reflects the broadest possible set of events. This is particularly important in volatile markets where outliers can significantly impact the covariance matrix.
Understanding the risk associated with illiquid assets is a fundamental aspect of financial analysis. Illiquid assets, such as real estate or private equity, are not frequently marked to market, which can lead to underestimation of their variance if based solely on returns. Therefore, it’s crucial to estimate the risk of these asset classes either from first principles or by adjusting risk differentials to baseline estimates of risk for stocks and bonds, considering leverage.
For example, let’s consider a private equity fund, like Blackstone, that invests in emerging, growth-oriented firms, financed half with debt and half with equity, using 2:1 leverage. The risk of an investment in their portfolio can be estimated by multiplying the risk of a small growth stock (say, 40%) by the leverage employed (2×). This results in a volatility of 80% for any given investment in the private equity fund.
If the fund has 16 independent investments, and all the investments are equally sized, the volatility of the fund can be calculated by dividing the volatility of each investment by the square root of the number of investments. However, in reality, investments are not equally sized and independent, and the correlations between them must be taken into account. This calculation provides a way to estimate the volatility of a private equity fund that is not traded on an exchange.
Understanding risk is crucial in financial management. Two key measures of risk are variance and covariance. These measures are popular due to their closed-form expression for portfolio variance, which is based on the variances and covariances of the portfolio’s assets. For instance, in a portfolio consisting of stocks from Apple and Microsoft, the variance would measure the volatility of each stock, while the covariance would measure how these stocks move in relation to each other.
Risks are not always apparent and can sometimes only be identified in hindsight. A prime example of this is the 2008 financial crisis, where AAA-rated mortgage securities, once considered virtually risk-free, turned out to be worthless, revealing the hidden risks obscured by unrealistic models and assumptions.
Effective wealth managers view risk from multiple dimensions. They assess the impact of various unfavorable scenarios on their clients’ portfolios and identify conditions where their baseline risk view fails. For example, during the 2008 financial crisis, wealth managers who had identified the hidden risks in mortgage securities could have adjusted their clients’ portfolios to minimize losses.
Portfolio Optimization and Asset Allocation are key components in wealth management. They involve the creation of a portfolio that aligns with a client’s objectives and risk tolerance, based on established capital market assumptions. This is typically achieved using Markowitz’s mean-variance optimization formulation.
The mean-variance optimization formulation is represented as:
$$\max \limits_w \left( \text{Portfolio expected return} – \frac{\gamma}{2} \times \text{Portfolio variance} \right)$$
Where:
The coefficient \(\gamma\) represents the client’s balance preference between reward (the first term) and variance (the second term). A small \(\gamma\) indicates a preference for high returns over low variance, while a large \(\gamma\) signifies a preference for low variance over high returns.
According to Levy and Markowitz (1979), \(\gamma\) can be selected to closely approximate most utility functions mentioned in economic literature. Psychologists’ discussions with investors suggest that \(\gamma\) is unlikely to fall below 1 or exceed 10, with a typical value being 3.
The mean-variance formulation is a key concept in portfolio construction, which often lacks explicit constraints. These constraints, such as long-only positions, limitations on leverage, maximum and minimum exposures to asset classes, sectors, and geographies, and turnover and trading constraints, play a significant role in the investment process.
Investors can use any preferred risk measure in the optimization process. However, computations involving risk measures other than those associated with mean-variance optimization tend to be more complex. For instance, if the risk measure cannot be computed using available data, a simulation may be required.
Simulations, while realistic and accommodating a wide range of constraints, tend to run more slowly than an optimization process. They are best suited to solving problems with 100 or fewer assets and asset classes.
The portfolio construction process involves creating a recommended allocation of the client’s portfolio and underlying investments. This process is often demonstrated through real-world case studies involving wealth managers and private clients.
Asset Liability Modeling and Surplus Management is a vital component of wealth management. It requires a comprehensive understanding of a client’s assets and liabilities, extending beyond mere asset-only optimizations. This knowledge is important for effective financial planning. In instances where clients do not maintain or regularly update their personal financial statements, the wealth manager assumes the responsibility of compiling these statements.
For families with assets in multiple countries, the wealth manager must be conversant with the laws governing investments in each relevant country. This understanding is crucial to determine the suitability and eligibility of each investment for each family member.
On a client’s personal balance sheet, assets typically include cash, deposit, and brokerage accounts, retirement accounts, employee benefits, ownership interests in private businesses, cash-value life insurance, real property, and personal assets. Liabilities typically include consumer debt, automobile loans, student loans, property-related loans, and margin debt in brokerage accounts.
Net worth, equity, discretionary wealth, or surplus refers to the difference between the total value of all assets and the total sum of all liabilities. For instance, if a client has assets worth $1 million and liabilities of $200,000, their net worth or surplus would be $800,000. Clients typically provide this information to wealth managers through account statements and tax filings.
Human capital, or the present value of future earnings, is not included in this balance sheet. For example, the McCarthys’ human capital is not included because their career trajectories and lifestyle choices are not specified. Therefore, only their financial assets and liabilities as well as their known spending plans are specified.
Practice Questions
Question 1: A private wealth client is considering various types of investments to optimize their portfolio. They are aware that the surplus, the difference between their assets and liabilities, plays a crucial role in their investment decisions. Which of the following statements is most accurate?
- The complexity of modeling returns for private investments is primarily due to their base fees.
- The risk of private investments is more challenging to estimate than their returns.
- Capital market expectations, which consider the impact of taxes on returns, are used as inputs in the construction of optimal portfolios.
Answer: Choice C is correct.
Capital market expectations, which consider the impact of taxes on returns, are indeed used as inputs in the construction of optimal portfolios. This is because the after-tax return is the actual return that the investor will receive, and it is this return that should be maximized. Taxes can significantly impact the net return of an investment, and therefore, they must be considered when forming capital market expectations. These expectations, which include estimates of the expected returns, risks, and correlations of different asset classes, are crucial inputs in the portfolio optimization process. They help determine the optimal allocation of assets in the portfolio that will maximize the investor’s expected utility, given their risk tolerance and investment horizon.
Choice A is incorrect. While it is true that the fee structures of private investments can be complex, this is not the primary reason why modeling returns for these investments is challenging. The main challenge lies in the lack of transparency and liquidity, the unique characteristics of each investment, and the difficulty in obtaining reliable data. The base fees are just one component of the total fees, which may also include performance fees, carried interest, and other costs.
Choice B is incorrect. The statement that the risk of private investments is more challenging to estimate than their returns is not accurate. Both the risk and return of private investments can be challenging to estimate due to the reasons mentioned above. However, it is not necessarily more difficult to estimate the risk than the return. The risk of an investment is typically estimated based on the variability of its returns, and if the returns are difficult to estimate, so is the risk.
Question 2: A wealth manager is making investment decisions for a client based on a client questionnaire approach. However, the manager is aware of the high degree of subjectivity in this approach as pointed out by academic studies. What is a potential consequence of this subjectivity in the client questionnaire approach?
- The wealth manager’s own views on risk may significantly influence the investment decisions for the client.
- The wealth manager may not be able to accurately assess the client’s risk tolerance.
- The wealth manager may not be able to provide personalized investment recommendations to the client.
Answer: Choice B is correct.
The potential consequence of the subjectivity in the client questionnaire approach is that the wealth manager may not be able to accurately assess the client’s risk tolerance. The client questionnaire approach is a common method used by wealth managers to understand a client’s investment objectives, risk tolerance, and financial situation. However, this approach is highly subjective and depends on the client’s understanding and interpretation of the questions. This can lead to inaccurate or inconsistent responses, making it difficult for the wealth manager to accurately assess the client’s risk tolerance. This can result in investment decisions that do not align with the client’s actual risk tolerance, potentially leading to unsatisfactory investment outcomes for the client. Therefore, it is crucial for wealth managers to supplement the client questionnaire approach with other methods to accurately assess a client’s risk tolerance.
Choice A is incorrect. While the wealth manager’s own views on risk may influence the investment decisions for the client, this is not a direct consequence of the subjectivity in the client questionnaire approach. The wealth manager’s views on risk should be based on objective analysis and should not be influenced by the subjective responses of the client.
Choice C is incorrect. The client questionnaire approach is designed to provide personalized investment recommendations to the client. While the subjectivity in this approach may lead to inaccuracies in assessing the client’s risk tolerance, it does not necessarily mean that the wealth manager will not be able to provide personalized investment recommendations. The wealth manager can still provide personalized recommendations based on the information obtained from the questionnaire, although these recommendations may not accurately reflect the client’s actual risk tolerance.
Private Wealth Pathway Volume 1: Learning Module 4: Investment Planning; LOS 4(a): Recommend and justify portfolio allocations and investments for a private client